Distributional-based texture classification using non-parametric statistics
Pattern Analysis and Applications(2007)
摘要
Texture classification is an important problem in image analysis. In the present study, an efficient strategy for classifying texture images is introduced and examined within a distributional-statistical framework. Our approach incorporates the multivariate Wald–Wolfowitz test (WW-test), a non-parametric statistical test that measures the similarity between two different sets of multivariate data, which is utilized here for comparing texture distributions. By summarizing the texture information using standard feature extraction methodologies, the similarity measure provides a comprehensive estimate of the match between different images based on graph theory. The proposed “distributional metric” is shown to handle efficiently the texture-space dimensionality and the limited sample size drawn from a given image. The experimental results, from the application on a typical texture database, clearly demonstrate the effectiveness of our approach and its superiority over other well-established texture distribution (dis)similarity metrics. In addition, its performance is used to evaluate several approaches for texture representation. Even though the classification results are obtained on grayscale images, a direct extension to color-based ones can be straightforward.
更多查看译文
关键词
Texture classification,Non-parametric distance,Distributional metric,Statistical graph matching,Texture features
AI 理解论文
溯源树
样例
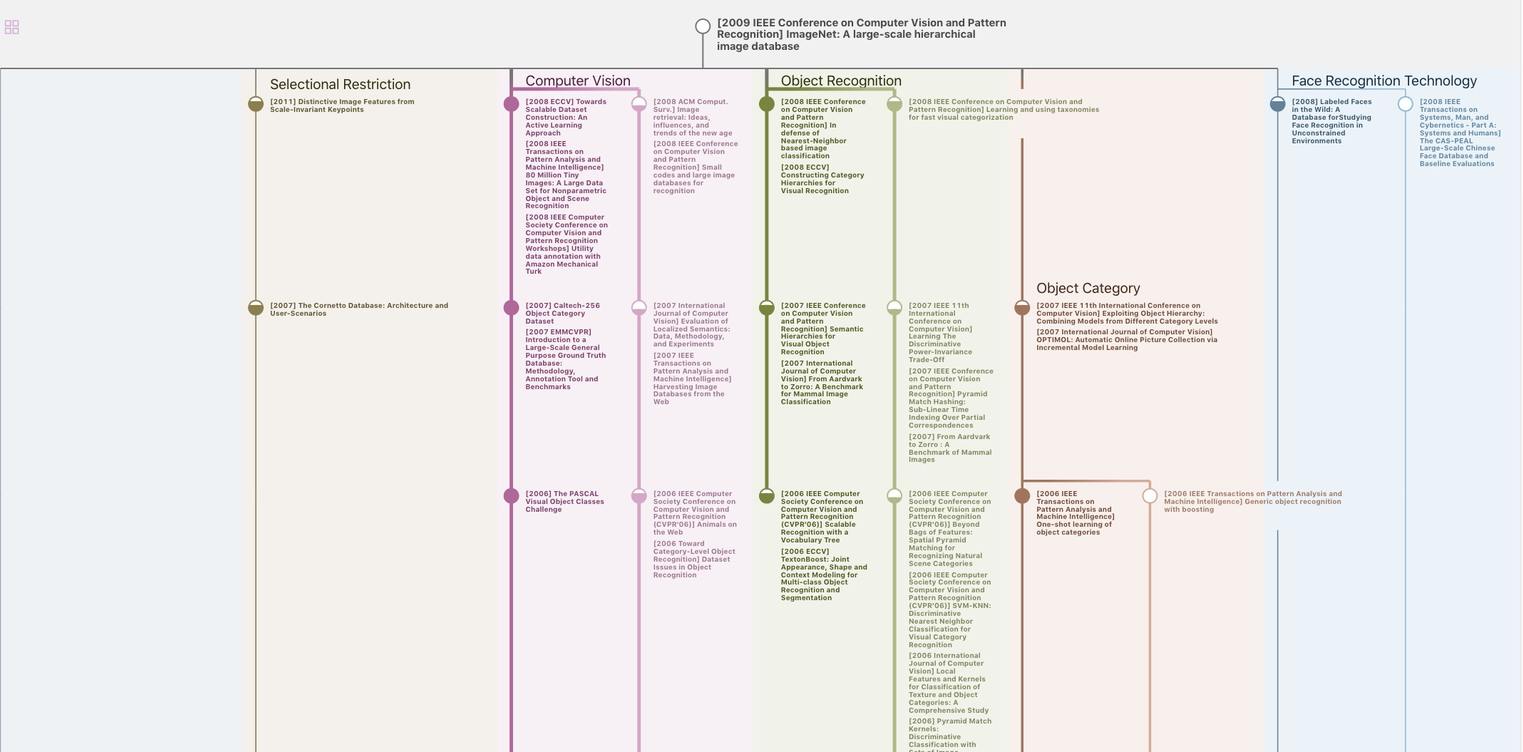
生成溯源树,研究论文发展脉络
Chat Paper
正在生成论文摘要