Provably good sampling and meshing of Lipschitz surfaces
SCG '06: Proceedings of the twenty-second annual symposium on Computational geometry(2006)
摘要
In the last decade, a great deal of work has been devoted to the elaboration of a sampling theory for smooth surfaces. The goal was to ensure a good reconstruction of a given surface S from a finite subset E of S. The sampling conditions proposed so far offer guarantees provided that E is sufficiently dense with respect to the local feature size of S, which can be true only if S is smooth since the local feature size vanishes at singular points.In this paper, we introduce a new measurable quantity, called the Lipschitz radius, which plays a role similar to that of the local feature size in the smooth setting, but which is well-defined and positive on a much larger class of shapes. Specifically, it characterizes the class of Lipschitz surfaces, which includes in particular all piecewise smooth surfaces such that the normal deviation is not too large around singular points.Our main result is that, if S is a Lipschitz surface and E is a sample of S such that any point of S is at distance less than a fraction of the Lipschitz radius of S, then we obtain similar guarantees as in the smooth setting. More precisely, we show that the Delaunay triangulation of E restricted to S is a 2-manifold isotopic to S lying at bounded Hausdorff distance from S, provided that its facets are not too skinny.We further extend this result to the case of loose samples. As an application, the Delaunay refinement algorithm we proved correct for smooth surfaces works as well and comes with similar guarantees when applied to Lipschitz surfaces.
更多查看译文
关键词
delaunay triangulation,finite subset e,smooth surface,smooth setting,piecewise smooth surface,similar guarantee,provably good sampling,singular point,lipschitz radius,local feature size,lipschitz surface,computer graphic
AI 理解论文
溯源树
样例
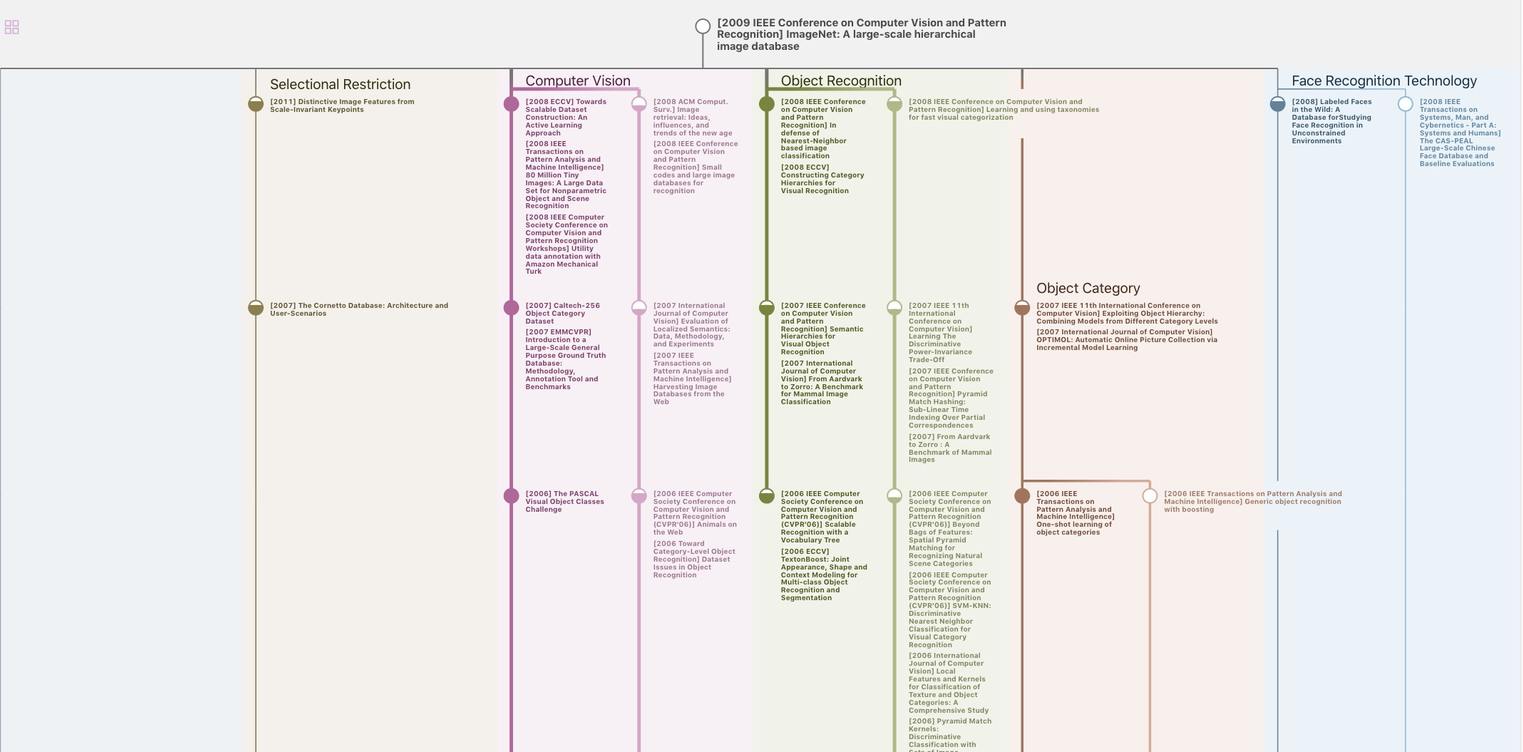
生成溯源树,研究论文发展脉络
Chat Paper
正在生成论文摘要