Comparing Machine Learning Classification Schemes ― a GIS Approach
ICMLA(2005)
摘要
This project examines the effectiveness of two classification schema: Support Vector Machines (SVM), and Artificial Neural Networks (NN) when applied to geographic (i.e. spatial) data. The context for this study is to examine patterns of urbanization in Mahoning County, OH in relation to several independent driving variables of urban development. These independent variables were constructed using Geographic Information Systems (GIS) and were compared to the dependent variable of the spatial locations of urban areas in Mahoning County. The classification techniques were used in conjunction with the GIS-created variables to predict the location of urban areas within Mahoning County. A comparison of the accuracy of the techniques is presented and conclusions drawn concerning which of the variables are the most influential on urban patterns in the region. Lastly, a spatial analysis of the prediction error is performed for each method.
更多查看译文
关键词
spatial analysis,mahoning county,independent variable,machine learning classification schemes,urban area,urban development,gis approach,classification technique,independent driving variable,spatial location,classification schema,urban pattern,spatial data,geographic information systems,machine learning,geography,artificial neural network,support vector machines,prediction error,geographic information system,support vector machine,learning artificial intelligence,neural nets
AI 理解论文
溯源树
样例
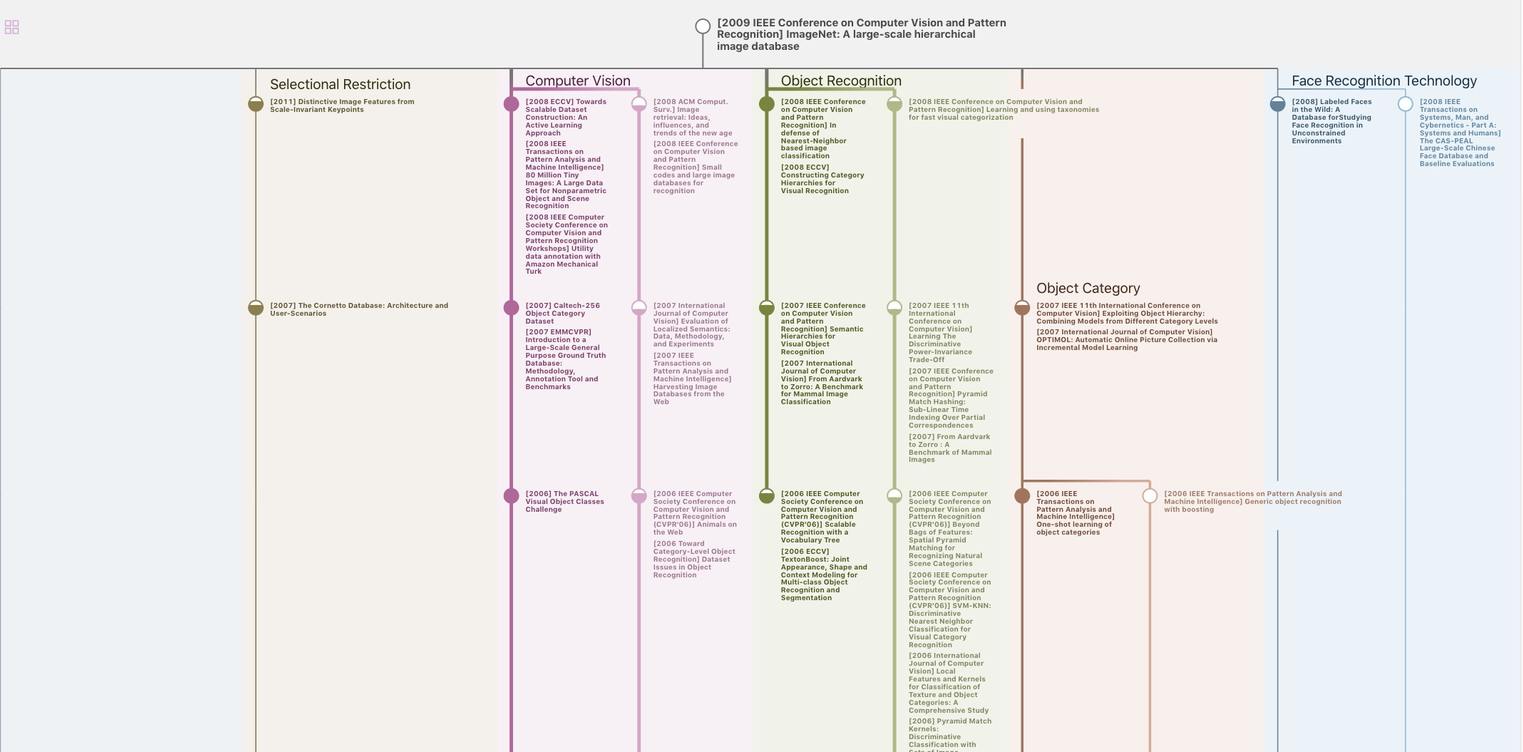
生成溯源树,研究论文发展脉络
Chat Paper
正在生成论文摘要